Partners: Wageningen Environmental Research
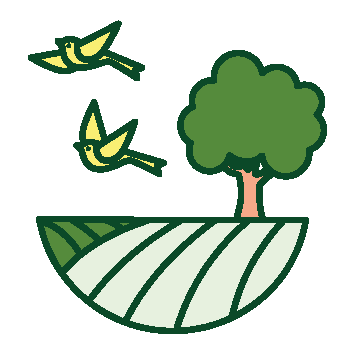
Objective
The main aim of Use Case 2 is to investigate the impact of agricultural activities on the environment (e.g., soil, groundwater, emissions etc.) and further on biodiversity. Within the use case customized machine learning tools are utilized within data cube-based infrastructure. The expected results are established workflows and data pipelines including a prototype of a model that predicts causal relations between changes in farmland bird biodiversity and specific agricultural practices in the Netherlands.
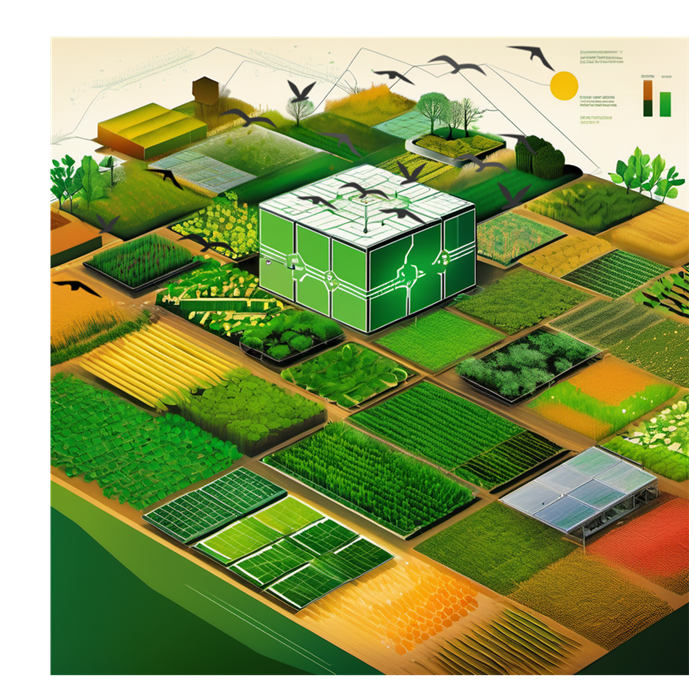
Applications
The established model can be used by decision makers in agriculture and environmental protection by supporting better-informed decisions such as selecting more nature-inclusive practices promoting biodiversity through specific applications:
- Spatial categorization: The results of the observation and estimation steps for biodiversity can be used to categorize agricultural landscapes and e.g. administrative regions, based on predicted suitability.
- Casual modeling: Causal modelling allows reasoning about potential situations to answer ‘What-if?” type of questions and creating scenarios for farmland landscape development considering biodiversity favorable conditions.
- Smart tools: The presented approach aims at improved understanding of causalities between farm activities and changes in biodiversity. When results are sufficiently robust, the model could be incorporated into advisory tools for farmers or policy makers, to help assess the consequences of actions.
Approach
Three main data categories (biodiversity, environment and agriculture) are handled primarily within their individual processing flows and data cubes, which are then ultimately merged using causal machine learning. Modelling methods such as causal inference and discovery provide insights into the underlying mechanisms describing the impact of agricultural practices on biodiversity. They do not only statistically predict the correlations but also provide meaningful explanations for those predictions, enhancing the overall interpretability of the model results.
All the tools are expected to be provided within FAIRiCUBE hub as shared data infrastructure and documented[1][2].
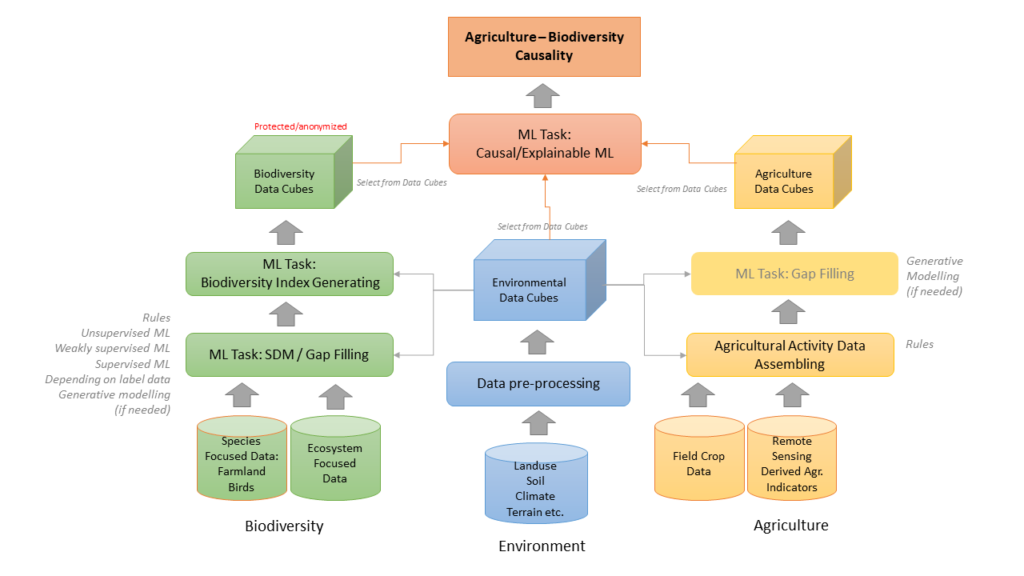
[1] https://github.com/FAIRiCUBE
[2] https://fairicube.readthedocs.io/en/latest/