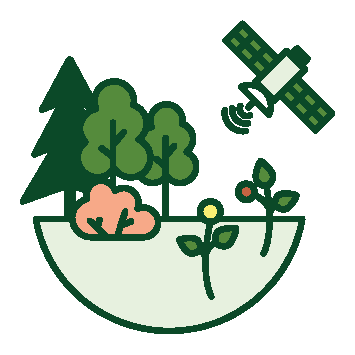
Partner: Naturhistorisches Museum Wien, Natural History Museum Vienna (NHMW)
Research problem
Systems of habitat classification are an important tool in nature conservation. Usually, they classify habitats based on an artificial classification system which can be also paired with vegetation units occurring. However, these methods ignore taxa occurrences and environmental factors for the classification.
Use Case objective
Use Case 5 aims to enhance the conventional method used in habitat classification by using machine learning to identify environmental factors influencing vegetation communities and their presence in the habitats. The final goal of the use case is to implement a method that could improve the current classification system of the European Habitats.
Workflow
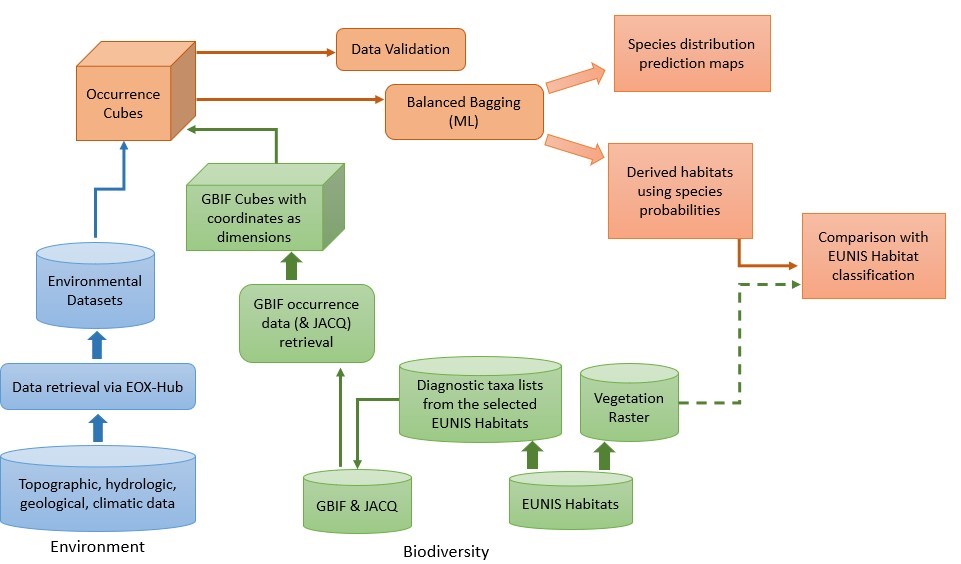
Habitats are selected from the European Nature Information System (EUNIS). The relative diagnostic taxa are used to retrieve the occurrence data from the Global Biodiversity Information Facility (GBIF). These data are first validated and checked for quality and then ingested as Occurrence Cubes.
Environmental data are chosen based on their relevance on the occurrence of taxa and obtained from satellite data through EOX services. These are then combined with the occurrence data to produce Extended Occurrence Cubes which will be used in the Machine Learning approaches.
In the machine Learning steps we use the Bootstrap aggregating strategy to predict the occurrence of taxa based on occurrence and environmental data. Lastly, we compare the predictions of diagnostic taxa of chosen habitats with the raster of EUNIS habitat types to check of overlaps and discrepancies.
Our method will allow us to investigate communities distribution along environmental gradients and to determine locations with favorable environmental conditions and taxa associations in more realistic habitat types.